Left Behind
One feature common to the AIs in this feature is the way they improve by learning. A robot at Imperial College London takes this idea a step further and is at the forefront of machine learning.
It’s able to formulate hypotheses, execute experiments, analyse the results and then decide what the follow-up experiment should be based on these results. It can then formulate a new hypothesis, and carry out that experiment too.
Stephen H Muggleton, head of Imperial College London’s Computational Bioinformatics Laboratory, described its work on the robot scientist in leading science publication ‘Nature’.
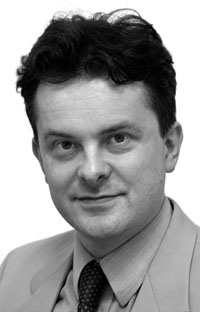
Professor Stephen Muggleton
"
Laboratory robots conducted experiments on yeast (saccharomyces cerevisiae) using a process known as 'active learning'. The aim was to determine the function of several gene knockouts by varying the quantities of nutrient provided to the yeast," Muggleton explained. "
The robot used a form of inductive logic programming (ILP) to select experiments that would discriminate between contending hypotheses."
This may leave you wondering what the point is of lowly mortals intervening in the process at all. As computers are able to process data around a million times faster than a human brain, we’re at a stage where we can be left behind, as Muggleton confirmed. "
The robot strategy that worked best – the lowest cost for a given accuracy of prediction – not only outperformed two other automated strategies, based on cost and random-experiment selection, but also outperformed humans given the same task."
However, Muggleton states in ‘Nature’ that it’s "
essential that developments in high-speed automation are not introduced at the expense of human understanding and insight".
We asked Muggleton if the possibility of being left behind by machines had ruffled the academic feathers of the science world.
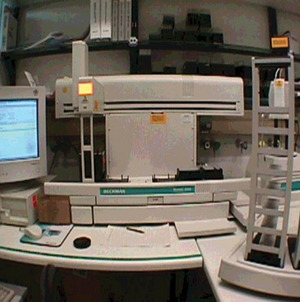
The Robot Science Project
"
There was some discussion of this issue in the editorial of the issue of “Nature” in which our paper appeared," he replied.
"
On balance, the editor saw the advantages of making science more efficient. I’ve had similar feedback from other senior scientists, and have yet to receive any highly negative responses. I suspect that this is partly to do with the fact that scientists find it easy to recognise the ubiquitous need for computational techniques that help to deal with the large data volumes present in all areas of science."
It’s one more example of a machine made by our own hands that can beat us at our own game. Arguably, it’s in one very specific area and the machine is simply doing the ‘donkey work’, in the same way that any machine in the home, such as a washing machine, automates tasks we once performed manually.
However, imagine creating a single machine and AI that could execute all these tasks. An AI that could drive, carry out experiments, play soccer and shark at poker, would have a formidable claim to resembling an intelligent being, especially if it were able, as a human is, to take lessons from one area and apply them to another.
Want to comment? Please log in.